Giles Hooker
V-Statistics and Variance Estimation:
Inference for Random Forests and Other Ensembles
June 1, 2023 at 16:00 CET
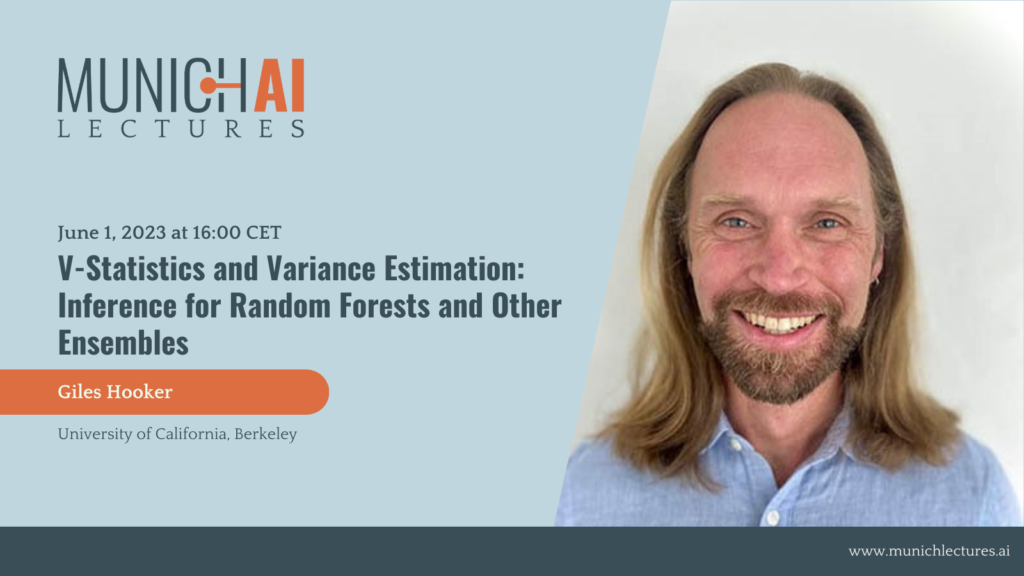
Abstract
This talk discusses uncertainty quantification and inference using ensemble methods. Recent theoretical developments inspired by random forests have cast bagging-type methods as U-statistics when bootstrap samples are replaced by subsamples, resulting in a central limit theorem and hence the potential for inference. However, to carry this out requires estimating a variance for which all proposed estimators exhibit substantial upward bias. In this talk, we convert subsamples without replacement to subsamples with replacement resulting in V-statistics for which we prove a novel central limit theorem. We also show that in this context, the asymptotic variance can be expressed as the variance of a conditional expectation which is approximated by sampling from the empirical distribution and allows for valid bias corrections. We finish by illustrating the use of these tools in combining or comparing statistical models.
Bio
Giles Hooker is a professor in the Department of Statistics at UC Berkeley. His research interests focus on the interface of statistics with both machine learning and applied mathematics, as well as functional data analysis and robust statistics. Much of his research has been inspired by applications in ecology and healthcare.
Join Us!
Please visit this site for more information on Zoom link for the talk.